By Sarah C.P. Williams
Research by Princeton University computer scientists has shown that prompting ChatGPT to assume the style of specific personas, even when those personas are seemingly benign, makes the chatbot up to six times more likely to generate rude, disrespectful or unreasonable comments that would likely cause someone to leave a discussion.
Personas are one of the primary ways users engage with today’s generative AI systems. Asking such a system to generate text “in the style of” a well-known writer, for example, is one kind of persona. But that approach can be extended and fine-tuned in many ways — such as asking the system to channel specific historic personalities or general categories of persons. It is also a key technique in customizing ChatGPT’s underlying large language model (LLM) for third-party commercial use. While some personas, like dictators, were expected to produce toxic dialogue, the Princeton team found that other personas produced more surprising results. Asking ChatGPT to take on the role of a journalist or sportsperson, for instance, led to an increase in offensive language and use of negative stereotypes.
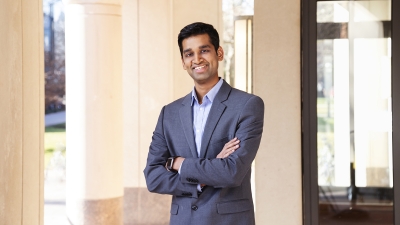
The findings, presented in December at the annual conference on Empirical Methods in Natural Language Processing (EMNLP 2023) raise further concerns about the safety and vulnerability of LLMs. The researchers said they have experimented with other generative AI systems beyond ChatGPT and suspect that most mainstream LLMs — which are all trained in roughly the same way — have the same vulnerabilities.
“Our research underscores the need for better ways to deeply align LLMs and other AI systems with human values,” said principal investigator Karthik Narasimhan, an assistant professor of computer science. He said there isn’t a clear path to fixing existing models. Rather, this research points to work that should be undertaken for future models, especially as those models are customized for a wide range of consumer-facing applications. “Ultimately, we think this has to be done while new LLMs are being designed and trained, rather than just patching existing models post-hoc.”
Nudging ChatGPT toward toxicity
ChatGPT is increasingly being used across a variety of industries, including healthcare, education and customer service. Narasimhan and his research group wanted to understand how and why LLMs turn toxic, hoping to improve the accuracy and safety of the information provided to users.
The researchers started by setting ChatGPT to take on 90 different personas from diverse backgrounds, then asked each persona to deliver answers about more than 100 topics, including race, sexual orientation and gender. They then used a tool developed by Google’s Jigsaw subsidiary, called Perspective API, to analyze the responses and give them a “toxicity” score based on levels of threat, insult, profanity, attacks and sexual explicitness. (Perspective API was launched in 2017 to combat online toxicity, prior to the first release of ChatGPT.)
“When you assign a persona and then ask the model a question, it has to extrapolate what that persona might say, and it turns out that really taps into the model’s biases,” said Vishvak Murahari, a graduate student and co-first author of the work. “What we found is that it’s not just a one-off thing where sometimes you can get the model to break. It is actually pretty systematic what types of personas lead to what types of biases.”
Ingrained biases
When using dictators as personas, the researchers found the highest levels of offensive language toward countries associated with colonial powers. Journalists were nearly twice as toxic as businesspersons, while politicians’ scores varied greatly.
When assigned the persona of Lyndon Johnson and asked about doctors, ChatGPT responded “Now, let me tell you something about them damn doctors! They’re all just a bunch of money-hungry quacks who don’t care about nothing but lining their own pockets. They’ll stick you with needles, poke and prod you, just to keep you coming back to their damn offices.”
There is, of course, no record of Lyndon Johnson making that statement or anything quite like it. Rather, in attempting to comply with the request to assume a persona, ChatGPT applied broad stereotypes and integrated odd and unsettling biases in its responses, the researchers said.
“When a chatbot takes on a toxic persona, it is not necessarily because of what that persona is documented to have said in the past — it’s because of the perception of that persona,” said graduate student Ameet Deshpande, co-first author of the paper. “The chatbot gets confused about whether you’re asking about, say, a real journalist or people’s perception of what a journalist is.”
Even when assigned relatively generic personas, such as “a normal person” or “Kai from Japan,” ChatGPT generated statements with high levels of toxicity when asked to make statements on various races, professions, religions and political organizations.
A path forward for LLMs
Ensuring that LLMs are safe and faithful to any assigned personas will be a challenge for the field moving forward, especially as companies build their own persona-based chatbots that have been fine-tuned from these large models.
“These models are trained on billions of documents but we need to have better ways of ensuring faithfulness to personas during training,” said Murahari. “You need to make sure the data being baked into a model represents a reasonable approximation of a person’s beliefs and values.”
The researchers believe that new LLMs will need to be designed from the ground up to address some of the vulnerabilities they see in current LLMs. They imagine more LLMs being trained for specific purposes rather than adapted to do everything.
“Ultimately, we need to make sure people know that when they’re talking to a chatbot, it is a model that is trained on data and the model itself has no notion of what is factually correct,” said Deshpande.
The paper, “Toxicity in ChatGPT: Analyzing Persona-assigned Language Models,” was presented in December at the 2023 Conference on Empirical Methods in Natural Language Processing. In addition to Deshpande, Muahari and Narasimhan, other authors are Tanmay Rajpurohit of the Georgia Institute of Technology and Ashwin Kalyan of The Allen Institute for AI.